FLUS Model Validation and Soil Utilization Forecast in Chiang Mai City and Surrounding Area
Keywords:
Land use, Physical factors, MARKOV, GeoSOS-FLUSAbstract
Land use represents the relationship of physical, social, political and technological factors. The objectives of this study are: 1) to study the land use change of the of Land Development Department in 2006, 2009 and 2015, in Amphoe Mueang Chiang Mai and surrounding areas, 2) to validate the FLUS Model and 3) to predict the land use change in 2024. The relationship of factors and land use by using Artificial Neuron Network (ANN) and the future scenario from Markov Model were applied to predict the land use change. The research results from land use change of Land Development Department in 2006 – 2009 revealed that agriculture area was decreased 34,453 rai, forest area was decreased 748 rai, miscellaneous area was increased 1,830 rai, built-up area was increased 29,922 rai and water bodies area was increased 3,449 rai. In addition, agriculture area was decreased 6,356 rai, forest area was decreased 2,460 rai, miscellaneous was decreased 5,648 rai, built-up area was increased 13,210 rai and water bodies was increased 1,254 rai in 2009-2015. According to land use change during 2006 – 2015, agriculture area was decreased 40,809 rai, forest area was decreased 3,208 rai, miscellaneous area was decreased 3,818 rai, built-up area was increased 43,132 rai and water bodies area was increased 4,703 rai. The land use change prediction in 2015 - 2024 illustrated that agriculture area was decreased 19,521 rai, forest area was decreased 6,268 rai, miscellaneous area was decrease 7,386 rai, built-up area was increased 35,013 rai and water bodies area was decreased 1,838 rai. The database can be implemented to land use management planning for Amphoe Mueang Chiang Mai and surrounding areas.
References
Ashraf, M. D., & Yamaguchi, Y. (2009). Land use and land cover change in Greater Dhaka, Bangladesh: Using remote sensing to promote sustainable urbanization. Applied Geography, 29(3), 390-401.
Brinkmann, k., Schumacher, J., Dittrich, A., Kadaore, I., & Buerkert, A. (2012). Analysis of landscape transformation processes in and around four West African cities over the last 50 years. Landscape and Urban Planning, 105(1-2), 94-105.
Chena, Y., Li, X., Liua, X., Ai, B., & Li, S. (2016). Capturing the varying effects of driving forces over time for the simulation of urban growth by using survival analysis and cellular automata. Landscape and Urban Planning, 152, 59–71.
Hyandye, C. & Martz, L. W. (2017). A Markovian and cellular automata land-use change predictive Model of the Usangu Catchment. International Journal of Remote Sensing, 38(1), 64-81.
Iamchuen, N. & Thepwong, W. (2020). Relationship between Physical Factors and Land Use for the Future Land Use Prediction. Journal of Architectural Planning Research and Studies. 17(2): 79-92.
Kiriwongwattana, K. (2007). Application of Clue-S Model for Land Use and Land Cover Changes Projection at Mae Yod Watershed, Maechaem District, Chiang Mai Province. Kasetsart University.
Khongouan, W. & Khamwachirapithak, P. (2020). Park Area Development Plan in Response to the Government Policies and Standards: Case Study of Krathumbaen Municipality, Samut Sakhon. Journal of Architectural/Planning Research and Studies. 17(2), 111-128.
Lambin, EF. et.al. (2001). The causes of land-use and land-cover change: Moving beyond the myths. Global Environmental Change. 11(4), 261–269.
Lanchanon, P. (2013). The Potential of Agricultural Lands for Conservation of Green Area in Chiang Mai City. Journal of Architectural/Planning Research and Studies. 10(2), 115-137.
Li, X. & Anthony, G. (2002). Urban Simulation Using Neural Networks and Cellular Automata for Land Use Planning. Springer-Verlag Berlin Heidelberg. 451-465.
Lianga, X., Liua, X., Lib, a. X., Chenb, Y., Tiana, H., & Yao, Y. (2018). Delineating multi-scenario urban growth boundaries with a CA-based FLUS Model and morphological method. Landscape and Urban Planning, 177, 47–63.
Liu, X., Li, X. & Liang, X. (2020). A Future Land Use Simulation Model by coupling Human and Natural Effects. GeoSOS-FLUS User's Manual.
Muhammad, H. S. & Han, S. L. (2019). Prediction of Land Use and Land Cover Changes for North Sumatra, Indonesia, Using an Artificial-Neural-Network-Based Cellular Automaton. Sustainability, 11(11), 1-16.
Pumchan, S., Trisuratand, Y. & Pipatwattanakul, D. (2014). Application of clue – s model and globio 3 model in the assess of land use change on biodiversity. Kasetsart University.
Ruensukon, T., Trisurat, Y., Tangtham, N., & Tokrisna, R. (2016). Valuation of ecosystem services in Klongchumphon watershed, Ranong and Chumphon province. Thai Journal of Forestry (Thailand).
Suppawimut, W. (2018). Agricultural land losses in surrounded area of chiang mai middle ring road. VRU Research and Development Journal Science and Technology. 13(3),103-113.
Thepwong, W. & Chakaew, N. (2019). Application on spatial models for land use prediction. The 4th Conference on Natural Resources, Geoinformation and Environment. 455-461.
Turner, II B.L., Skole, D., Sanderson, S., Fischer, G., Fresco, L. & Leemans, R. (1995). Land use land cover change. science/research plan (IGBP Report No. 35 and HDP Report No.7).
Vanno, S. (2012). Bangkok’s Green Infrastructure. Journal of Architectural/Planning Research and Studies. 9(2), 1-13.
Wang, Y., Xu, T., Lv, X., & Zhang, Z. (2016). Simulation of Land Use Dynamic Change using selected driving factors based on the method of Feature Selection. Advances in Engineering Research, 3, 855-860. 35(1), 62-73.
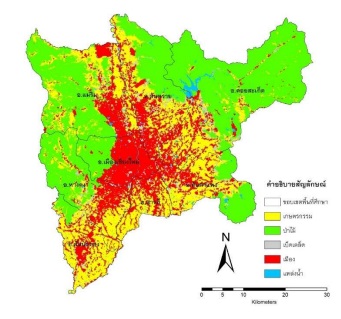