Recognition of Multiple Power Quality Disturbances Based on Discrete Wavelet Transform and Improved Long Short-Term Memory Networks
DOI:
https://doi.org/10.69650/rast.2024.255814Keywords:
Recognition, Multiple Power Quality Disturbances, Discrete Wavelet Transform, Improved Long Short-Term Memory NetworksAbstract
In the past few years, distributed electricity generation from renewable sources, or microgrid systems, has been connected to the grid to increase power supply stability. This responds to government policy regarding commitment to using 100% renewable energy in operations (RE100) project efforts. This results in the entry of power electronic or non-linear equipment into the electrical system, making it more sensitive. Moreover, multiple power quality disturbances (PQDs) consist of a variety of single disturbances. Analysis of complex multi-label patterns is a challenging task. In this paper, we propose a methodology to address this challenge by leveraging Discrete Wavelet Transform (DWT) and Improved Long Short-Term Memory Networks (LSTM). Firstly, multiple PQDs are synthesized utilizing a mathematical model based on IEEE standards 1159-2019. Secondly, the obtained PQDs are decomposed into nine feature classes, yielding detailed (cDs) and approximation (cAs) coefficients through Five-Level DWT Decomposition. Furthermore, we conducted a comparative analysis of each component across five different wavelet functions: haar1, db4, bior1.3, coif2, and sym4. Thirdly, the cDs and cAs coefficients derived from each wavelet type undergo statistical analysis before being inputted into the LSTM model for classification of each feature class. Our results highlight that cD5 components obtained from the db4 wavelet exhibit the highest accuracy rate of 93.86%. This finding elucidates the significance of selecting appropriate wavelet types and compositions for the successful classification of multiple PQDs.
References
Chung, I. Y., Won, D. J., Kim J. M., Ahn S. J. and Moon S. I., Development of a network-based power quality diagnosis system. Electric Power Systems Research. 77(8) (2007) 1086–1094, doi: https://doi.org/10.1016/j.epsr.2006.09.011.
Mohanty, S.R., Ray P. K., Kishor N. and Panigrahi B. K., Classification of disturbances in hybrid DG system using modular PNN and SVM. International Journal of Electrical Power & Energy Systems. 44(1) (2013) 764–777, doi: https://doi.org/10.1016/j.ijepes.2012.08.020.
Khetarpal, P. and Tripathi, M. M., A critical and comprehensive review on power quality disturbance detection and classification. Sustainable Computing Informatics and Systems. 28 (2020) 400-417, doi: https://doi.org/10.1016/j.suscom.2020.100417.
IEEE Recommended Practice for Monitoring Electric Power Quality, IEEE Std 1159-2019 (Revision of IEEE Std 1159-2009), 2 (2019) 1-98, doi: https://doi.org/10.1109/IEEESTD.2019.8796486.
Provincial Electricity Authority, Regulations on electrical networksSystem connection requirements, <https://www.pea.co.th/Portals/0/Document/vspp/PEA%20Interconnection%20Code%202016.pdf> (2016).
Provincial Electricity Authority, Power quality research department, research division, Electrical System Research and Development Department, <https://www.pea.co.th.> (2023).
Saxena, D., Verma, K. S. and Singh, S., Power quality event classification: an overview and key issues. International Journal of Engineering, Science and Technology. 2(3) (2010) 186-199, doi: https://doi.org/10.4314/ijest.v2i3.59190.
S. Upadhyaya and S. Mohanty, Power quality disturbance detection using wavelet-based signal processing. in Annual IEEE India Conference (INDICON. (2013) 1-6, doi: https://doi.org/10.1109/UPEC.2016.8114077.
Dekhandji, F. Z., Detection of power quality disturbances using discrete wavelet transform. in 5th International Conference on Electrical Engineering - Boumerdes (ICEE-B). (2017) 1-5, doi: https://doi.org/10.1109/ICEE-B.2017.8192080.
Diego, R. I. and Barros, J., Subharmonic measurement using DFT and wavelet-packet transform in an IEC extended framework, Measurement. 43 (2010) 1603–1608, doi: https://doi.org/10.1016/j.measurement.2010.09.012.
Pan, M. Xu, Chen, T., J. and Li, Z., A harmonic detection method for distributed generation connected grid system using DWT and HHT. Journal of Electrical Engineering & Technology. 14(4) (2019) 1495–1503, doi: https://doi.org/10.1007/s42835-019-00190-1.
Parvez, M., Aghili, A. I., Sarwat, S., Rahman, R. and Alam, F., Online power quality disturbance detection by support vector machine in smart meter. Journal of Modern Power Systems and Clean Energy. 7 (2018) 1328–1339, doi: https://doi.org/10.1007/s40565-018-0488-z.
Mahela, O. P., Khan, B., Alhelou, H. H., and Tanwar, S., Assessment of power quality in the utility grid integrated with wind energy generation. IET Power Electronics. 13(13) (2020) 2917–2925, doi: https://doi.org/10.1049/iet-pel.2019.1351.
Mahela, O. P., Khan, B., Alhelou, H. H and Siano, P., Power quality assessment and event detection in distribution network with wind energy penetration using stockwell transform and fuzzy clustering. IEEE Transactions on Industrial Informatics. 16(11) (2020) 6922–6932, doi: https://doi.org/10.1109/tii.2020.2971709.
Exploring Technologies. Discrete wavelet transform (DT) of 1-D Signals, <https://www.exptech.co.in/.> (2022).
Rico-Medina, V., Reyes-Archundia, E., Gnecchi, J. A. G., Olivares-Rojas, J. C. and Del-Carmen, M., Garcia-Ramirez, C., Analysis of the appropriate decomposition level based on discrete wavelet transform for detection of power quality disturbances. in IEEE International Autumn Meeting on Power, Electronics and Computing (ROPEC). (2022) 1-6, doi: https://doi.org/10.1109/ropec55836.2022.10018687
Chawda, G. S., Shaik, A. G., Shaik, M., Padmanaban, S., Nielsen, J. B. H., Mahela, O. P. and Kaliannan, P., Comprehensive review on detection and classification of power quality disturbances in utility grid with renewable energy penetration. IEEE Access. 8 (2020) 146807–146830, doi: https://doi.org/10.1109/access.2020.3014732.
Addison, P. The illustrated wavelet transform handbook, <https://doi.org/10.1887/0750306920> (2002).
Siddiqi, H. Applied functional analysis: numerical methods, Wavelet methods, and image processing. 1st edn, CRC Press, 2003.
Semmlow, J. L., Biosignal and biomedical image processing: MATLAB-based applications, <http://ci.nii.ac.jp/ncid/BA66341356> (2022).
Cui, J., Gao, Q. and Li, D., Improved long short-term memory network based short term load forecasting. in 2019 Chinese Automation Congress (CAC). (2019), 4428-4433, doi: https://doi.org/10.1109/CAC48633.2019.8996379.
Karasu, S. and Sarac, Z., Classification of power quality disturbances by 2D-Riesz transform, multi-objective grey wolf optimizer and machine learning methods. Digital Signal Processing. 101 (2020) 102711, doi: https://doi.org/10.1016/j.dsp.2020.102711.
MathWorks. MATLAB version: 9.13.0 (R2022b], <https://www.mathworks.com> (2023).
Janthong, S. and Phukpattaranont, P., Classification of double power quality disturbances using discrete wavelet transform and bi-directional recurrent neural networks. in PEACON & Innovation 2023: Moving towards to digital grid and green energy, Bangkok Thailand. (2023), 50-59.
Zhang, B., Chen, H., Feng, G. and Zhang, H., Application of wavelet multi-resolution analysis to harmonics detection based on MATLAB in power system. in IEEE International Conference on Automation and Logistics. (2007), 137-143, doi: https://doi.org/10.1109/ICAL.2007.4338545.
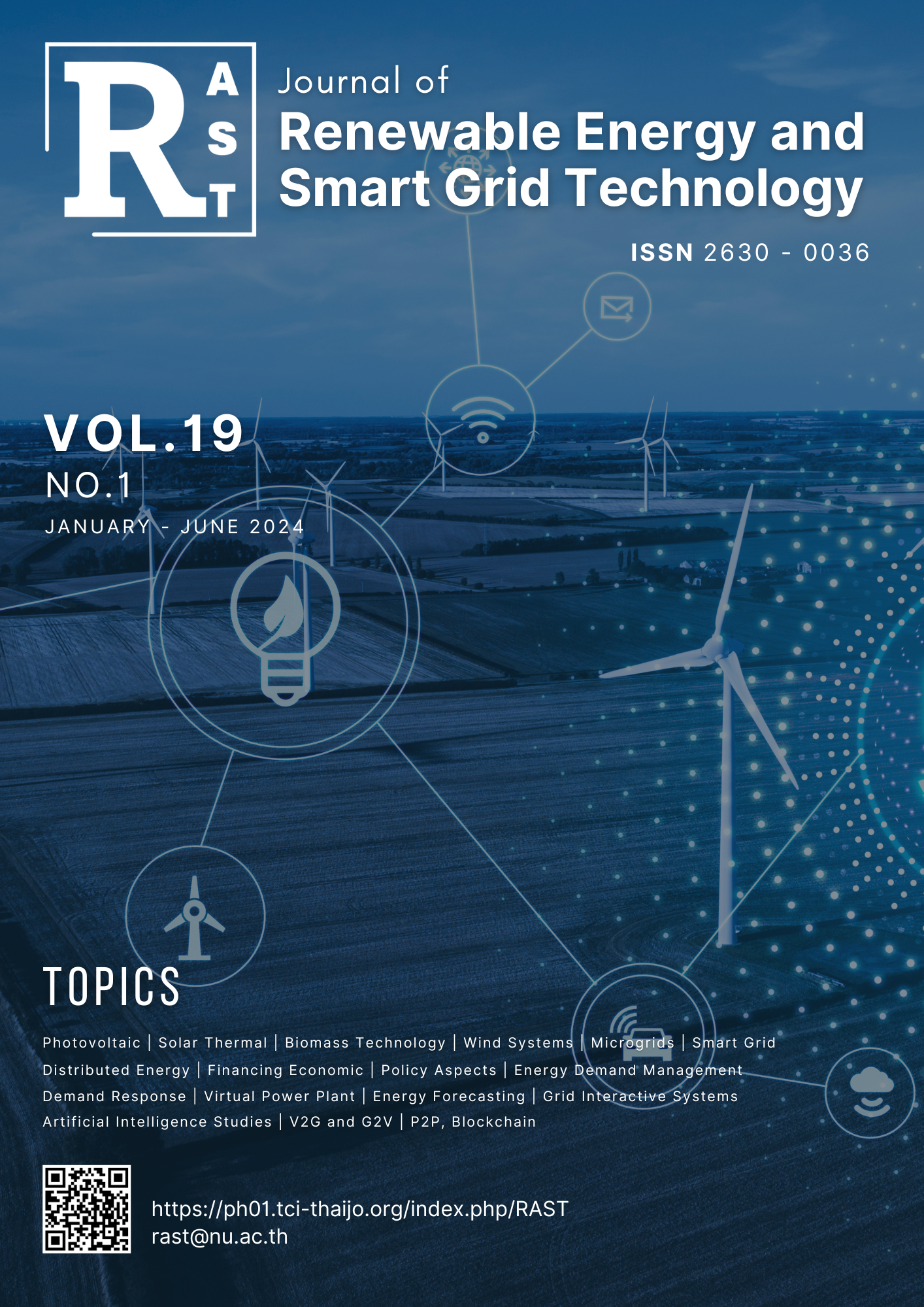
Downloads
Published
How to Cite
Issue
Section
License
Copyright (c) 2024 School of Renewable Energy and Smart Grid Technology (SGtech)

This work is licensed under a Creative Commons Attribution-NonCommercial-NoDerivatives 4.0 International License.
All copyrights of the above manuscript, including rights to publish in any media, are transferred to the SGtech.
The authors retain the following rights;
1. All proprietary rights other than copyright.
2. Re-use of all or part of the above manuscript in their work.
3. Reproduction of the above manuscript for author’s personal use or for company/institution use provided that
(a) prior permission of SGtech is obtained,
(b) the source and SGtech copyright notice are indicated, and
(c) the copies are not offered for sale.