A Comparison of Future Simulation of Land Use Models in Urbanization A Case Study of Buriram District, Buriram Province
Keywords:
Land use model, CA-Markov, Land Change Modeler, BuriramAbstract
Buriram Province It is one of the most rapidly expanding provinces in Thailand. from the fact that there is a railway line that cuts through the middle of the city. It is an economic city, a sports city, and a tourism city. This led to an interest in studying the development of Buriram city using a comparative land use model. The purpose is to validate the model. And to forecast land use in the year 2032 by using CA-Markov (CAM) and Land Change Modeler 3 methods, which are Multi-Layer Perceptron (MLP), Logistic Regression (LR) and SimWeight (SM). And a total of 10 factors were included in the analysis, namely 1) Digital elevation model 2) Slope 3) Aspect 4) Distance from the municipality 5) Distance from local government 6) Distance from road 7) distance from railways, 8) stream, 9) soil drainage, and 10) population density. The results showed that area trends during 2007-2012 and 2012-2022, building area and water source area are increasing, but agricultural land and forest areas has reduced. After that checking the land use model validation, it was found that in the overall accuracy method and the Kappa coefficient, the MLP, LR, and SM methods higher accuracy than the CAM method. The 2032 land use forecast found that all four methods yielded quantitatively similar results. (Area), but there are differences in terms of the land use allocation with concepts that result in different maps. This output can be applied to different situations and contexts in different areas.
References
กรมการปกครอง. (2565). ระบบสถิติทางการทะเบียน (Official statistics registration systems). ส่วนบริหารและพัฒนาเทคโนโลยีการทะเบียน สำนักบริหารการทะเบียน กรมการปกครอง. สืบค้นวันที่ 20 กรกฎาคม 2565. https://stat.bora.dopa.go.th/stat/statnew/statMenu/newStat/sumyear.php/
กลุ่มงานยุทธศาสตร์และข้อมูลเพื่อการพัฒนาจังหวัด. (2563). แผนพัฒนาจังหวัดบุรีรัมย์ (ปี 2561-2565) ฉบับทบทวน ปี 2563. สำนักงานจังหวัดบุรีรัมย์.
เกศินี นงโพธ์, ชูเดช โลศิริ และ สุธาทิพย์ ชวนะเวสสกุล. (2564). การติดตามและการคาดการณ์การเปลี่ยนแปลงการใช้ประโยชน์ที่ดินในอนาคตจังหวัดอุดรธานี. วารสารสังคมศาสตร์ มหาวิทยาลัยศรีนครินทรวิโรฒ. 24(1): 13-34.
ณัฐวุฒิ บุตรคล้อ, มานัส ศรีวณิช และนิติ เอี่ยมชื่น. (2564). การเปลี่ยนแปลงการใช้ที่ดินและการขยายตัวของเมืองอุตสาหกรรม กรณีศึกษา อําเภอศรีมหาโพธิ จังหวัดปราจีนบุรี. การประชุมวิชาการ Built Environment Research Associates Conference ครั้งที่ 12 (BERAC 12) 28 มิถุนายน 2564. ปทุมธานี: มหาวิทยาลัยธรรมศาสตร์. 408-418.
นงนภัส ชวนกำเนิดการ (2563). ภาพฉายการเปลี่ยนแปลงการใช้ประโยชน์ที่ดิน ปี ค.ศ. 2050 บริเวณพื้นที่ภาคตะวันออกของประเทศไทย ด้วยแบบจำลอง Land Change Modeler. ศิลปศาสตรบัณฑิต (ภูมิศาสตร์) คณะศิลปศาสตร์ มหาวิทยาลัยธรรมศาสตร์.
นิติ เอี่ยมชื่น และ วันณัชชา เทพวงศ์. (2563). การหาความสัมพันธ์ระหว่างปัจจัยทางกายภาพกับการใช้ประโยชน์ที่ดินเพื่อคาดการณ์การใช้ประโยชน์ที่ดินในอนาคต. วารสารวิจัยและสาระสถาปัตยกรรม/การผังเมือง. 17(2): 79-92.
มานัส ศรีวณิช, ชลิตา เถยศิริและนิติ เอี่ยมชื่น. (2561). Modeling Urban Growth and Analyzing Urban Spatial Patterns in the City of Suratthani, Thailand. Science Journal Chandrakasem Rajabhat University. 28(1), 18-24.
วุฒิพงษ์ นิลจันทร์ และ นิติ เอี่ยมชื่น. (2563). การคาดการณ์ปริมาณการใช้ไฟฟ้าภาคเหนือตอนบนของประเทศไทย. วารสารวิทยาศาสตร์บูรพา. 25(1): 76-89.
Alberg, A. J. Park, J. W. Hager, B. W. Brock, M. V and Diener-West, M. (2004) The Use of “Overall Accuracy” to Evaluate the Validity of Screening or Diagnostic tests. J Gen Intern Med. 19(5):460-465.
Bergsma, W. (2013). A bias correction for Cramer's V and Tschuprow's T. Journal of the Korean Statistical Society. 42(3): 323-328. DOI: 0.1016/j.jkss.2012.10.002.
Bhatti, S. S., Tripathi, N. K., Nitivattananon, V., Rana, I. A., & Mozumder, C. (2015). A multi-scale modeling approach for simulating urbanization in a metropolitan region. Habitat International. 50: 354-365.
Congalton, R. (1991). A review of assessing the accuracy of classifications of remotely sensed data. Remote Sensing of Environment. 37(1): 35-46.
Kolb, M. and Galicia, L. (2013). Scenarios and story lines: drivers of land use change in southern Mexico. Environ Dev Sustain. 20: 681–702.
Mishra, V. N., Rai, P. V. and Mohan, K. (2014). Prediction of land use changes based on land change modeler (LCM) using remote sensing: A case study of Muzaffarpur (Bihar), India. J. Geogr. Inst. Cvijic. 64(1): 111-127.
Sangermano, F. Eastman, J. R. and Zhu, H. (2010). Similarity Weighted Instance-based Learning for the Generation of Transition Potentials in Land Use Change Modeling. Transactions in GIS. 14(5): 569-580.
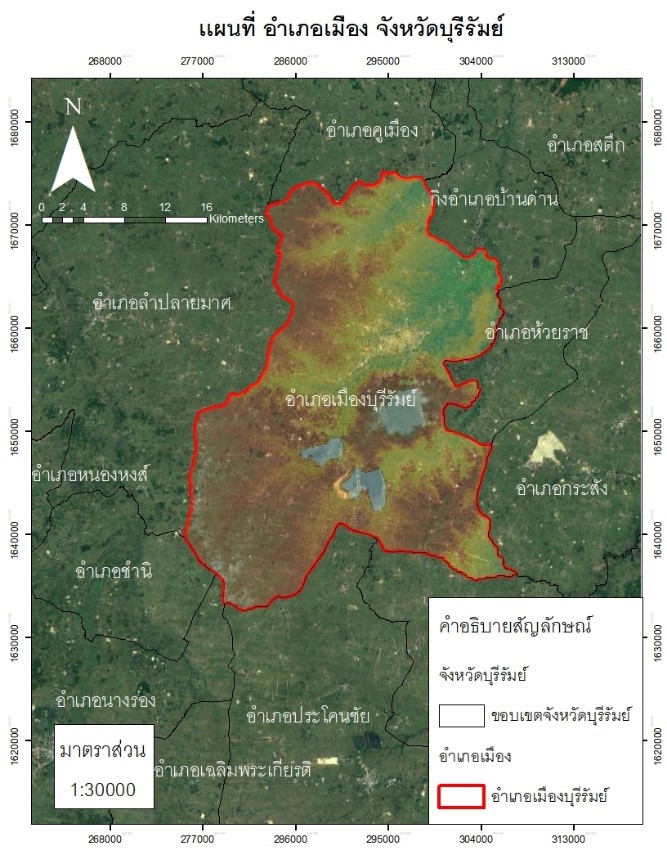