Evaluation consistency of high-resolution image derived from UAV and Sentinel-2 images for monitoring growth of rice
Keywords:
Vegetation Index, Vegetation Phenology, Unmanned Aerial VehicleAbstract
The purposes of this study were to monitor the growth of rice in the study area using high-resolution images derived from unmanned aerial vehicle (UAV) and Sentinel-2 Image with GEE and to calculate the vegetation indices including Excess Green (ExG), Visible Difference Vegetation Indices (VDVI), Green-Red Vegetation Indices (GRVI) and Normalized Differential Vegetation Indices (NDVI) in order to evaluate the consistency of vegetation Indices with simple linear regression and correlation coefficient between vegetation Indices derived the same sensor and cross sensor. The results showed that the vegetation phenology from both sensors tended to increase related to the growth of rice to the peak maximum greenness of rice and decrease since reduced greenness when harvest season. In addition, vegetation phenology derived from sentinel-2 fluctuated since weather barriers effects (cloud cover). In terms of vegetation Indices conformity derived from the same sensor, it illustrated the positive relations and high consistency which different from cross sensor (UAV and satellite) that some of vegetation Indices had low consistency. However, the findings indicated that UAV could be applied in conjunction with three vegetation indices to monitoring growth of rice.
References
กรมการข้าว. (2564). โครงการภายใต้ยุทธศาสตร์ข้าวไทย 2563-2567.
ประกาศโฆษณาการรับขึ้นทะเบียนสิ่งบ่งชี้ทางภูมิศาสตร์. (2565). กรมทรัพย์สินทางปัญญา กระทรวงพาณิชย์ (เล่ม 63 เลขที่ประกาศ 186, น. 1-4).
ปริญฉัตร จุนถาวร, กฤชญาณ อินทรัตน์ และ นิลันดอน ไสยะวง. (2564). การเปรียบเทียบดัชนีพืชพรรณ RGB กับดัชนีพืชพรรณผลต่างแบบนอมัลไลซ์ ด้วยข้อมูลจากอากาศยานไร้คนขับ กรณีศึกษาข้าวแปลงเล็ก. การประชุมวิชาการ (Proceedings) ทรัพยากรธรรมชาติ สารสนเทศภูมิศาสตร์ และสิ่งแวดล้อม ครั้งที่ 5 (NatGen 5th), วันที่ 6 – 7 พฤษภาคม 2564 (น. 50-59). คณะวิทยาการสารสนเทศ มหาวิทยาลัยมหาสารคาม.
สำนักงานส่งเสริมการค้าในต่างประเทศ ณ กรุงสิงคโปร์/ สำนักงานส่งเสริมการค้าในต่างประเทศ ณ กรุงมะนิลา. (2562). ภาพรวมตลาดข้าวในอาเซียน. สืบค้นจาก https://www.ditp.go.th/contents_attach/564288/564288.pdf สืบค้นจาก https://www.esa.int/Enabling_Support/Operations/Sentinel-2_operations
European Space Agency. (2022). Sentinel-2 operations.
Kalisperakis, I., Stentoumis, C., Grammatikopoulos, L., & Karantzalos, K. (2015). Leaf area index estimation in vineyards from UAV hyperspectral data, 2D image mosaics and 3D canopy surface models. The International Archives of Photogrammetry, Remote Sensing and Spatial Information Sciences, 40(1), 299.
Mangewa, L. J., Ndakidemi, P. A., Alward, R. D., Kija, H. K., Bukombe, J. K., Nasolwa, E. R., & Munishi, L. K. (2022). Comparative Assessment of UAV and Sentinel-2 NDVI and GNDVI for Preliminary Diagnosis of Habitat Conditions in Burunge Wildlife Management Area, Tanzania. Earth, 3(3), 769-787.
Soesanto, O., Idris, M., & Hastomo, H. D. (2022). Segmentasi vegetasi lahan basah berbasis modified-camera drone. In Prosiding Seminar Nasional Lingkungan Lahan Basah. 7(1), 259-266.
Soontranon, N., Lawawirojwong, S., Jitkajornwanich, K., Srestasathiern, P., & Rakwatin, P. (2017). A comparison of ground and satellite based phenologies for monitoring rice field. Bangkok: Geo-Informatics and Space Technology Development Agency (GISTDA).
Soontranon, N., Srestasathiern, P., & Rakwatin, P. (2015, June). Rice crop calendar based on phenology analysis from time-series images. In 2015 12th International Conference on Electrical Engineering/Electronics, Computer, Telecommunications and Information Technology (ECTI-CON) (pp. 1-5). IEEE.
Tucker, C. J. (1979). Red and photographic infrared linear combinations for monitoring vegetation. Remote sensing of Environment, 8(2), 127-150.
Woebbecke, D. M., Meyer, G. E., Von Bargen, K., & Mortensen, D. A. (1995). Shape features for identifying young weeds using image analysis. Transactions of the ASAE, 38(1), 271-281.
Zhou, H., Fu, L., Sharma, R. P., Lei, Y., & Guo, J. (2021). A hybrid approach of combining random forest with texture analysis and VDVI for desert vegetation mapping Based on UAV RGB Data. Remote Sensing, 13(10), 1891.
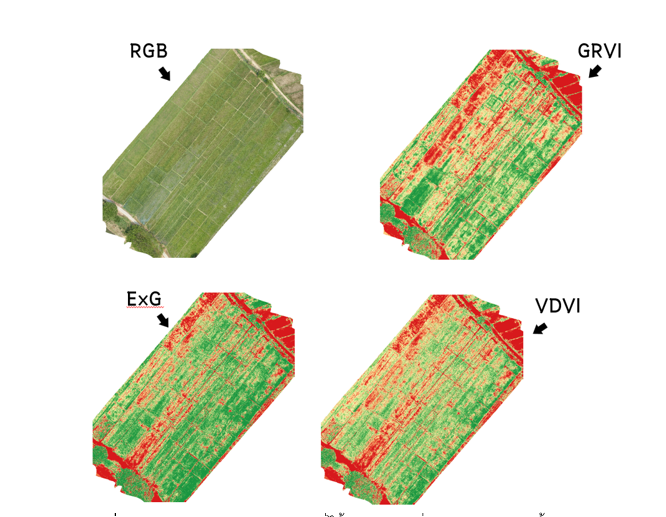