A Study on Support Vector Machine Image Classification: A Case Study on Rice Plantation Area in Chun District, Phayao Province
Keywords:
Support Vector Machine, Maximum Likelihood, Image classification, Paddy fieldAbstract
This research aims to study the performance of Support Vector Machine (SVM) using Landsat 8 satellite imagery to identify the paddy field in Chun District, Phayao Province. Training point and the checkpoint for classification accuracy assessment were developed from the Land Use Data Set from the Land Development Department and field surveys. Training point consists of 6 levels including 25, 50, 75, 100, 125 and 150 points. Supervised classification using SVM and Maximum Likelihood Classification (MLC) was compared by considering the statistical values; overall accuracy and Kappa coefficient. The overall accuracy values from SVM are reported at a high level of 89.66%, 89.90%, 90.89, 91.63%, 93.60%, and 94.33% respectively. And The overall accuracy values are reported at 83.25%, 83.50%, 86.21%, 87.19%, 85.96% and 86.95% respectively when MLC are applied. The Kappa coefficient values from SVM are also reported at a high level of 0.79, 0.80, 0.82, 0.83, 0.87, and 0.89, respectively. And the kappa are reported at 0.67, 0.67, 0.72, 0.74, 0.72, and 0.74 respectively when MLC are in use. According the results, it was found that SVM is more effective than MLC to identify the paddy field of the study area. It is hope that the method proposed in the study can be applied to other similar image classification.
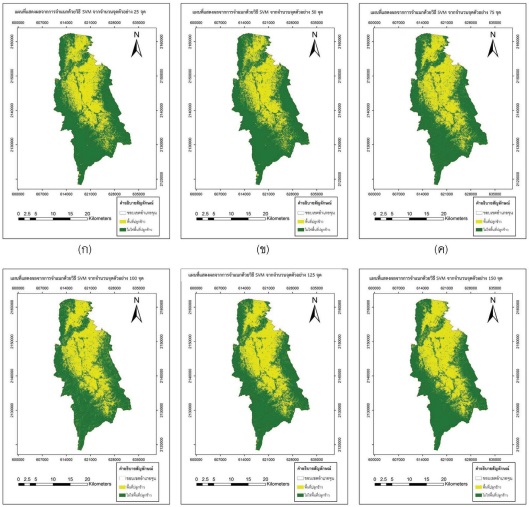