A Comparison of Forecasting Models of Stock Price using ARIMA and GVM Models
Keywords:
ARIMA model, Grey Model, GVM Model, Mean Absolute Percentage Error, Root Mean Square ErrorAbstract
The objective of this study is to construct the appropriate forecasting model for the BBL Stock Price via the use of Box-Jenkins method and Grey models. The daily average data, which were collected during July 2022 to June 2023 of 240 days were divided into 2 datasets. The first dataset, which consisted of 222 months from July 2022 to May 2023 was used for constructing the forecasting models via the use of 3 statistical methods, namely, Box-Jenkins, GM(1,1) and Grey Verhulst Model (GVM) methods. The second dataset, which consisted of 18 days from June 2023 was used for comparing the accuracy of the forecasting model. The criteria of the lowest mean absolute percentage error (MAPE) and root mean squared error (RMSE) were used for comparing the accuracy of the forecasting model. Research findings indicated that for all forecasting methods that were studied, the most accurate method was the GM(1,1) method. This forecasting method was 0.724 percent forecasting error (MAPE = 0.724) or was forecasting error of 1.463 (RMSE = 1.463). The comparison of the accuracy of the forecasting model for both criteria provided the same result. Therefore, it made more reliable that the GM (1,1) method was the most suitable method for this time series.
References
Kayacan, E., Ulutas, B., & Kaynak, O. (2010). Grey system theory-based models in time series prediction.Expert systems with applications,37(2), 1784-1789.
Shen, C., Du, F., Zhang, L., & Song, H. (2019, February). Application of GM (1, N) model in groundwater mineralization in Cheng’an County. InIOP Conference Series: Earth and Environmental Science, 233(4), 042010. IOP Publishing.
Puripat, C., & Sarikavanij, S. (2018). The comparison of Grey system and the Verhulst model for rainfall and water in Dam prediction.Advances in Meteorology,2018.
Davis, P. D., Amankwah, G., & Fang, Q. (2019). Predicting the rainfall of ghana using the grey prediction model GM (1, 1) and the Grey verhulst model.International Research Journal of Engineering and Technology (IRJET),6(8), 1362-1372.
He, F., & Tao, T. (2014). An Improved Coupling Model of Grey-System and Multivariate Linear Regression for Water Consumption Forecasting.Polish Journal of Environmental Studies,23(4).
Amphanthong, P., & Busababodhin, P. (2015). Forecasting PM10 in the upper northern area of Thailand with grey system theory.Burapha Science Journal,20(1), 15-24.
Doungdee, N., & Chiangpradit, M. (2018). Forecasting Crisis Peaks of Economics in Thailand Using Grey Model.Burapha Science Journal, 1111-1122.
Liu, S., & Forrest, J. Y. L. (2010).Grey systems: theory and applications. Springer Science & Business Media.
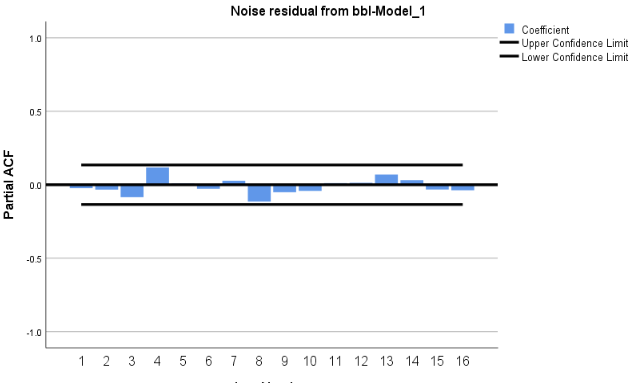
Downloads
Published
License
Copyright (c) 2023 Journal of Applied Science and Emerging Technology

This work is licensed under a Creative Commons Attribution-NonCommercial-NoDerivatives 4.0 International License.