Confidence Interval for the Population Mean of Rayleigh Distribution with Application to Shear wave Velocity of Soils in Thailand
Keywords:
Interval Estimation, Continuous Distribution, Coverage probability, Generalized Confidence IntervalAbstract
Rayleigh distribution is one of continuous probability distributions applied in several areas, especially in engineering and medicine. This probability model is also widely occurred in natural phenomenon. Since Rayleigh distribution is related to qualitative data, we are interested in studying interval estimation for the population mean. The two methods based on Wald-type and generalized confidence intervals are used in construction. Then, the performance of the proposed confidence intervals is conducted using simulations in terms of coverage probability and expected length. The results illustrate that the novel generalized confidence interval performs better than the compared confidence intervals in all cases in the study. Finally, a real data set on the shear wave velocity of soils in Lamphun province of Thailand is used in computation, in order to confirm the results from simulation.
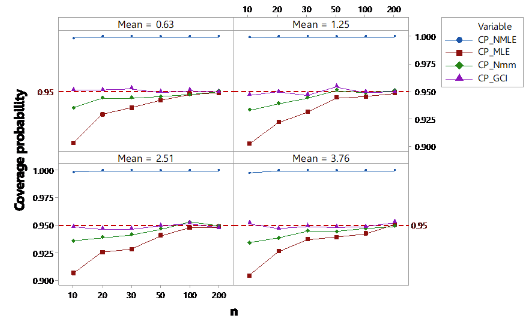
Downloads
Published
License
Copyright (c) 2023 Journal of Applied Science and Emerging Technology

This work is licensed under a Creative Commons Attribution-NonCommercial-NoDerivatives 4.0 International License.