Generative Adversarial Network for DOTA2 Character Generation
Keywords:
Generative adversarial networks, deep learning, DOTA2, StyleGAN, Few-shot image generationAbstract
DOTA2 is a popular game in the E-sport game industry with a high market value. In particular, if developing a variety of in-game character traits, this can increase the company's revenue and player satisfaction. Many studies can demonstrate the effectiveness of Generative Adversarial Networks (GAN) applications to create cartoon images or recreate portraits. Thus, this research aims to study the effectiveness of the Style-GAN application to be used in recreating and redesigning the DOTA2 character. The form of creating a small number of shots through a few-shot Image Generation via Cross-domain Correspondence, which has the distinction of not requiring the large number of images used in training as a regular GAN and not overfitting with too much accuracy. The dataset used in training was the thumbnail of the DOTA2 hero character, with a size of 256x144 pixels, 111 images, and made up of 3 models: 1) the Source_ffhq model (a StyleGAN2 model that learned to create human faces), 2) the Church models (StyleGAN2 models that learned to create church images), and 3) the Horse models (a StyleGAN2 model that learned to create horses). Quantitative comparisons showed that models adapted from the FFHQ model could correctly adapt a person's eyes and nose to the character's eyes and nose. However, the mouth part was erased, and other models could only create half the image but still correspond to the character traits. The images created by the three models were quite dark and fresh, which also looked like the images of a DOTA2 hero. In the Fréchet Inception Distance (FID) metric results, images created with a low FID score were as close as the original DOTA2-style images. As for qualitative evaluation, it was found that the sample of players have preferred the Source_ffhq model the most, followed by Church models and Horses models, respectively. The main factors were decided by being familiar with DOTA2. Therefore, this research can guide the creation of new character images from the original DOTA2 hero character series, with the opportunity to increase revenue for the game developer company, player satisfaction, and knowledge of further game development using GAN techniques.
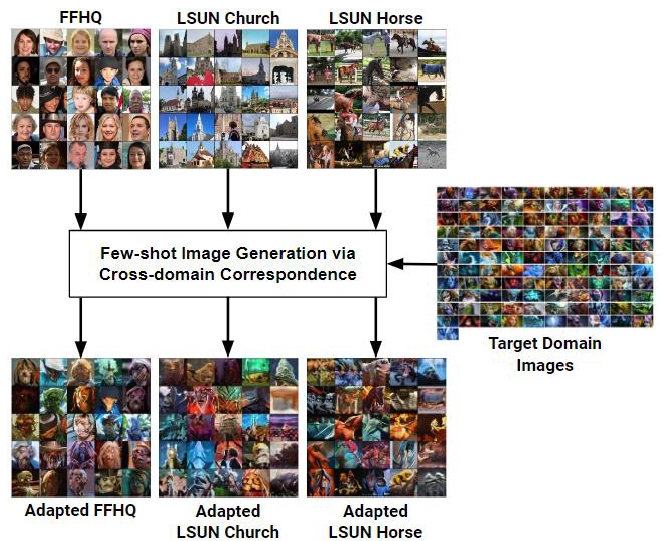
Downloads
Published
Issue
Section
Categories
License
Copyright (c) 2023 Journal of Applied Science and Emerging Technology

This work is licensed under a Creative Commons Attribution-NonCommercial-NoDerivatives 4.0 International License.