Application of a Long Short - Term Memory Deep Natural Network Model for Field Crops Price Forecasting in Thailand
DOI: 10.14416/j.ind.tech.2022.12.013
Keywords:
Field crops prices, Long short-term memory (LSTM), Artificial neural network model (ANN), Hybrid modelAbstract
The objective of this research was to select a forecasting model suitable for the time series data of three sets of field crop prices, namely cassava, corn and paddy rice prices. Two-hundred and forty-one values were collected from the website of the Office of Agricultural Economics, Ministry of Agriculture and Cooperatives from January 2002 to January 2022. Three forecasting models were constructed: an artificial neural network model (ANN), a long short-term memory (LSTM) deep neural network model and a hybrid model. The models’ accuracy was compared by three performance evaluations: mean absolute error (MAE), root mean square error (RMSE) and mean absolute percent error (MAPE). The results showed that the hybrid model provided better forecast accuracy than the ANN model and the LSTM model in all data sets and all forecasting performance criteria. It can be concluded that the hybrid model was suitable for forecasting the time series data of three sets of field crop prices.
References
K. Thongmeethip, Agricultural development in Thailand in terms of community development and quality of life, PSDS Journal of Development Studies, Puey Ungphakorn School of Development Studies, Thammasat University, 2021, 4(1), 132-162. (in Thai)
http://www.nso.go.th (Accessed on 20 March 2022)
https://data.worldbank.org (Accessed on 20 March 2022)
Office of agricultural economics, Agricultural statistics of Thailand 2020, Office of agricultural economics, Ministry of Agriculture and Cooperatives, Bangkok, Thailand, 2021.
https://www.oae.go.th (Accessed on 21 March 2022)
W. Riansut and K. Thongrit, Forecasting the price of field corn in Thailand, RUMTP Research Journal, 2017, 11(1), 1-14. (in Thai)
S. Deepradit and P. Rukson, The forecasting techniques comparison of field crops in
Thailand, The Journal of Industrial Technology, 2021, 17(3), 214 – 231. (in Thai)
T. Xiong, C. Li, and Y. Bao, Seasonal forecasting of agricultural commodity price using a hybrid STL and ELM method: Evidence from the vegetable market in China, Neurocomputing, 2018, 275, 2831-2844.
T. Iizumi, Y. Shin, W. Kim, M. Kim and J. Choi, Global crop yield forecasting using
seasonal climate information from a multi-model ensemble, Climate Services, 2018, 11, 13-23.
S.Y. Han, T.F.A. Bishop and P. Filippi, Data-driven, early-season forecasts of
block sugarcane yield for precision agriculture, Field Crops Research, 2022, 276, 108360.
T. Sujjaviriyasup, Artificial neural network model for forecasting monthly price of maize in Thailand, Srinakharinwirot Science Journal, 2018, 34(1), 91-107. (in Thai)
H. Yin, D. Jin, Y.H. Gu, C.J. Park, S.K. Han and S.J. Yoo, STL-ATTLSTM: Vegetable price forecasting using STL and attention mechanism-based LSTM, Agriculture, 2020, 10(12), 612.
N. Filipović, S. Brdar, G. Mimić, O. Marko and V. Crnojević, Regional soil moisture prediction system based on Long Short-Term Memory network, Biosystems Engineering, 2022, 213, 30-38.
J. Zhang, Y. Meng, J. Wei, J. Chen and J. Qin, A novel hybrid deep learning model for sugar price orecasting based on time series decomposition, Mathematical Problems in Engineering, 2021, 6507688.
L. Yu, S. Liang, R. Chen and K.K. Lai, Predicting monthly biofuel production using a hybrid ensemble forecasting methodology, International Journal of Forecasting, 2022, 38(1), 3-20.
H. Rezaei, H. Faaljou and G. Mansourfar, Stock price prediction using deep learning
and frequency decomposition, Expert Systems with Applications, 2021, 169, 114332.
B. Gao, X. Huang, J. Shi, Y. Tai and J. Zhang, Hourly forecasting of solar irradiance based on CEEMDAN and multi-strategy CNN-LSTM neural networks, Renewable Energy, 2020, 162, 1665-1683.
W. Liu, W.D. Liu and J. Gu, Forecasting oil production using ensemble empirical model decomposition based Long Short-Term Memory neural network, Journal of Petroleum Science and Engineering, 2020, 189, 107013.
Y.-X. Wu, Q.-B. Wu and J.-Q. Zhu. Improved EEMD-based crude oil price forecasting using LSTM networks, Physica A: Statistical Mechanics and Its Applications, 2019, 516, 114-124.
X. Yan, Y. Liu, Y. Xu and M. Jia, Multistep forecasting for diurnal wind speed based on hybrid deep learning model with improved singular spectrum decomposition, Energy Conversion and Management, 2020, 225, 113456.
J. Cao, Z. Li and J. Li, Financial time series forecasting model based on CEEMDAN and LSTM, Physica A: Statistical Mechanics and Its Applications, 2019, 519, 127-139.
Y. Dong, C. Zhang, M. Niu, S. Wang and S. Sun, Air pollution forecasting with multivariate interval decomposition ensemble approach, Atmospheric Pollution Research, 2021, 12(12), 101230.
N.E. Huang, Z. Shen, S.R. Long, M.C. Wu, H.H. Shih, Q. Zheng, N.C. Yen, C.C. Tung and H.H. Liu, The empirical mode decomposition and the Hilbert spectrum for nonlinear and nonstationary time series analysis, Proceedings of the Royal Society A: Mathematical, Physical and Engineering Sciences, 1998, 454(1971), 903–995.
N. Kulvanich, Improving prediction accuracy of time series data using ARIMA-ANN hybrid model and regression analysis, Thai Science and Technology Journal, 2020, 28(12), 2101-2112. (in Thai)
M. Niu, S. Sun, J. Wu, L. Yu and J. Wang, An innovative integrated model using the singular spectrum analysis and nonlinear multi-layer perceptron network optimized by hybrid intelligent algorithm for short-term load forecasting, Applied Mathematical Modelling, 2016, 40(5-6), 4079-4093.
G.P. Zhang, Time series forecasting using a hybrid ARIMA and neural network model, Neurocomputing, 2003, 50, 159-175.
Y. Lin, Y. Yan, J. Xu, Y. Liao, and F. Ma, Forecasting stock index price using the CEEMDAN-LSTM model, The North American Journal of Economics and Finance, 2021, 57, 101421.
http://colah.github.io/posts/2015-08-Understanding-LSTMs/ (Accessed on 18 March 2022)
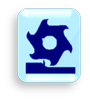
Downloads
Published
Issue
Section
License

This work is licensed under a Creative Commons Attribution-NonCommercial-NoDerivatives 4.0 International License.
ผลงานวิจัยและบทความวิชาการที่ปรากฏในวารสารนี้ เป็นความคิดเห็นอิสระของผู้เขียน ผู้เขียนจะต้องเป็นผู้รับผิดชอบต่อผลทางกฎหมายใด ๆ ที่อาจจะเกิดขึ้นจากบทความนั้น กองบรรณาธิการและคณะจัดทำวารสารฯไม่จำเป็นต้องเห็นด้วยเสมอไป