K-means Clustering for Grouping Product Size for Reducing Cost Packaging
DOI: 10.14416/j.ind.tech.2020.07.003
Keywords:
K-means Clustering, Design of Packaging Box Size, Box Compression TestAbstract
The brake pad industry has many packaging box sizes resulting in 3 disadvantages: (1) the high purchasing cost; purchasing each packaging box size per time with low volume, (2) a lot of storage space, and (3) low transport volume; there is no clear standard and the box's load limit is unknown. Therefore, the research aimed to group the products for designing the box sizes and find the most layers that can be stacked on a pallet. In the case of the study is to propose packaging design guidelines, to reduce purchase costs, reduce storage space and increase transport volume. This work implemented in 2 main methods. (1) K-mean clustering applied to group the products for designing a new package box size, which received in 3 sets of data, 437 data sets each. (2) analyzing the number of products that can be stacked on the pallet by applied the Box compression test (BCT) with the factors that affect the quality of the box. As a result, from proposing to 8 package design guidelines and evaluate the internal factor evaluation matrix method. The 5th approach is the most suitable guideline for the company's needs. It is designed horizontal size of packaging two sizes without adding foam and canceling sizes with foam. These found the cost reduced by 2.9 million baht or 13.43%. The storage space reduced by 2.19 million cubic inches or 48.65% and the transport volume increased by 5,200 boxes/round or 86.7%
References
[2] L.V. Bijuraj (2013) Clustering and its Applications, National Conference on New Horizons in IT–NCNHIT, Proceedings, 169-172.
[3] S. Nattaporn and R. Sunisa, K-Means Clustering of the Stock Exchange of Thailand 50 (SET 50) for Portfolio Diversification, Research Gate. 2013. (in Thai) Available on Research Gate: www.researchgate.net/publication/235907198_KMeans_Clustering_of_the_Stock_Exchange_of_Thailand_50_SET_50_for_Portfolio_Diversification (Accessed on 1 July 2020)
[4] C. Sujira, M. Parinya and P. Watchara (2017) An Application of K-Means for Candle Stick Clustering, Proceeding, RMUTP Business School, 2016. (in Thai)
[5] S. Panida, Performation Comparison of Data Mining’s Classification Method on Data Set with Outliers, Thai Science and Technology Journal (TSTJ), 2018, 27(6), 976-988.
[6] V. Kanlaya, Multivariate data analysis, 3rd Ed., Chulalongkorn University, Bangkok, TH, 2008. (in Thai)
[7] S. Ananthi and G. Thailambal, A Study on K-Means Clustering in Text Mining Using Python, International Journal of Computer Systems, 2016, 3(8), 560-564.
[8] J. Meng, H. Shang and L. Bian, (2009) The Application on Intrusion Detection Based on K-means Cluster Algorithm, International Forum on Information Technology and Applications, Proceeding, 150-152.
[9] P. Danai, Cluster Analysis, Academic Documents, Nation University, 2015.
[10] www.pt-pack.com/ กระดาษลูกฟูก/ การทดสอบกระดาษลูกฟูก.html. (Accessed on 10 June 2019)
[11] W. Sujin, Material Handling System Design, Academic Documents, Silpakorn University, 2017.
[12] C. Wichan, Performance improvement of package arrangement considering load bearing, Thesis, Chiang Mai University, 2013.
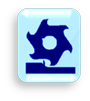