Comparative Analysis of Long Short-Term Memory and Random Forest Models for GULF Stock Closing Price Prediction
Keywords:
Stock Closing Price Prediction, Machine Learning, Deep Learning, Random Forest Model, Long Short-Term Memory ModelAbstract
In this study, we conducted a comparative analysis of the performance of a Random Forest model and a Long Short-term Memory model (LSTM) in predicting the closing price of GULF stock which has the characteristics of a growth stock. The data used in this study included closing and opening prices from March 21, 2018, to December 31, 2022, along with technical indicators such as EMA, TEMA, and WMA. Three comparative studies were conducted: the first case utilized a Random Forest model with optimal parameters, the second one utilized an LSTM model with optimal parameters, and the third case was similar to the second one but with a modification in the activation function from Hyperbolic Tangent function (Tanh) to Exponential Linear Unit function (ELU). The performance of these three models was evaluated based on the error values and the predicted value graphs. Our findings indicate that the Random Forest model had the lowest performance for GULF stock closing price prediction among the three cases. Conversely, the modified LSTM model with the ELU activation function in the third case demonstrated the highest performance among the three cases.
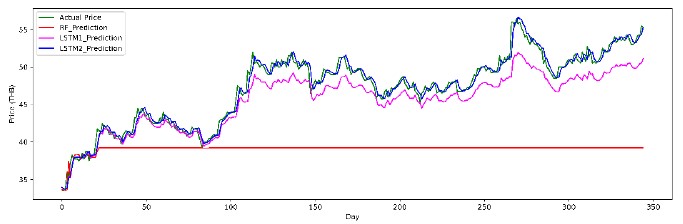
Downloads
Published
Issue
Section
Categories
License
Copyright (c) 2024 Journal of Applied Science and Emerging Technology

This work is licensed under a Creative Commons Attribution-NonCommercial-NoDerivatives 4.0 International License.