Vision-based Deep Learning for Anomaly Detection from Video Cartoon for Kids
Keywords:
Deep Learning, Video Analytics, Anomay Detection, Image Classification, Transfer LearningAbstract
According to the survey in 2020, Youtube is the most popular social media platform for Thai children in generation Z. Due to the overwhelming number of kid videos on Youtube, it is impossible for parent to check every single video and thoroughly look in every single image frame for any possible frame with inappropriate contents. To help ease this problem, this paper proposes using vision-based deep learning techniques to automatically detect frames with inappropriate visual content for children. In this work, our focus is on analyzing cartoon videos and the inappropriate content refers to blood scenes and weapons (gun and knife). To detect blood scenes, we use transfer learning and finetuning techniques with three pre-trained image classification backbone models—ResNet50, VGG16 and VGG19. Our experimental results show that VGG16 is the best that gives the highest accuracy of 0.84 and the highest area under ROC of 0.92. As for the part of weapon detection, we do transfer learning on two pre-trained object detection models, YOLOv3 and YOLOv4. Our results reveal that YOLOv3 is better in detecting gun and knife in cartoon videos.
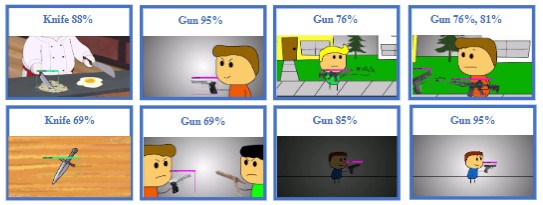
Downloads
Published
Issue
Section
Categories
License
Copyright (c) 2022 The Journal of Applied Science

This work is licensed under a Creative Commons Attribution-NonCommercial-NoDerivatives 4.0 International License.