Comparison of classification model for steam trap valve opening sound
Keywords:
sound classification, steam trap, support vector machine, long short-term memoryAbstract
This research aims to create models for steam trap valve opening sound classification by using two classification methods including support vector machine (SVM) and long short-term memory (LSTM). This study employs five feature extraction methods including zero-crossing rate, spectral centroid, Mel-frequency cepstral coefficient, spectral rolloff, and short-term Fourier transform. The results show that F1 score of SVM and LSTM are equivalent with a value of 66.67%. However, SVM provides higher precision than LSTM with value of 63.64% and 52.94%, respectively. In addition, LSTM gives higher recall than SVM with value of 90.00% and 70.00%, respectively.
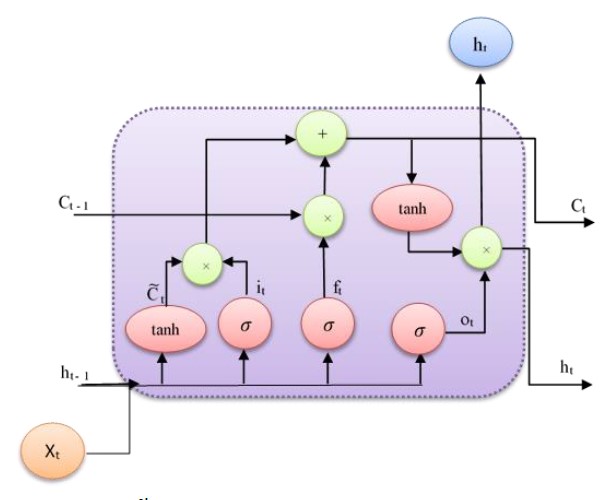
Downloads
Published
Issue
Section
Categories
License
Copyright (c) 2022 The Journal of Applied Science

This work is licensed under a Creative Commons Attribution-NonCommercial-NoDerivatives 4.0 International License.